6.1: Overview of Non-Experimental Research
- Page ID
- 16118
\( \newcommand{\vecs}[1]{\overset { \scriptstyle \rightharpoonup} {\mathbf{#1}} } \)
\( \newcommand{\vecd}[1]{\overset{-\!-\!\rightharpoonup}{\vphantom{a}\smash {#1}}} \)
\( \newcommand{\id}{\mathrm{id}}\) \( \newcommand{\Span}{\mathrm{span}}\)
( \newcommand{\kernel}{\mathrm{null}\,}\) \( \newcommand{\range}{\mathrm{range}\,}\)
\( \newcommand{\RealPart}{\mathrm{Re}}\) \( \newcommand{\ImaginaryPart}{\mathrm{Im}}\)
\( \newcommand{\Argument}{\mathrm{Arg}}\) \( \newcommand{\norm}[1]{\| #1 \|}\)
\( \newcommand{\inner}[2]{\langle #1, #2 \rangle}\)
\( \newcommand{\Span}{\mathrm{span}}\)
\( \newcommand{\id}{\mathrm{id}}\)
\( \newcommand{\Span}{\mathrm{span}}\)
\( \newcommand{\kernel}{\mathrm{null}\,}\)
\( \newcommand{\range}{\mathrm{range}\,}\)
\( \newcommand{\RealPart}{\mathrm{Re}}\)
\( \newcommand{\ImaginaryPart}{\mathrm{Im}}\)
\( \newcommand{\Argument}{\mathrm{Arg}}\)
\( \newcommand{\norm}[1]{\| #1 \|}\)
\( \newcommand{\inner}[2]{\langle #1, #2 \rangle}\)
\( \newcommand{\Span}{\mathrm{span}}\) \( \newcommand{\AA}{\unicode[.8,0]{x212B}}\)
\( \newcommand{\vectorA}[1]{\vec{#1}} % arrow\)
\( \newcommand{\vectorAt}[1]{\vec{\text{#1}}} % arrow\)
\( \newcommand{\vectorB}[1]{\overset { \scriptstyle \rightharpoonup} {\mathbf{#1}} } \)
\( \newcommand{\vectorC}[1]{\textbf{#1}} \)
\( \newcommand{\vectorD}[1]{\overrightarrow{#1}} \)
\( \newcommand{\vectorDt}[1]{\overrightarrow{\text{#1}}} \)
\( \newcommand{\vectE}[1]{\overset{-\!-\!\rightharpoonup}{\vphantom{a}\smash{\mathbf {#1}}}} \)
\( \newcommand{\vecs}[1]{\overset { \scriptstyle \rightharpoonup} {\mathbf{#1}} } \)
\( \newcommand{\vecd}[1]{\overset{-\!-\!\rightharpoonup}{\vphantom{a}\smash {#1}}} \)
\(\newcommand{\avec}{\mathbf a}\) \(\newcommand{\bvec}{\mathbf b}\) \(\newcommand{\cvec}{\mathbf c}\) \(\newcommand{\dvec}{\mathbf d}\) \(\newcommand{\dtil}{\widetilde{\mathbf d}}\) \(\newcommand{\evec}{\mathbf e}\) \(\newcommand{\fvec}{\mathbf f}\) \(\newcommand{\nvec}{\mathbf n}\) \(\newcommand{\pvec}{\mathbf p}\) \(\newcommand{\qvec}{\mathbf q}\) \(\newcommand{\svec}{\mathbf s}\) \(\newcommand{\tvec}{\mathbf t}\) \(\newcommand{\uvec}{\mathbf u}\) \(\newcommand{\vvec}{\mathbf v}\) \(\newcommand{\wvec}{\mathbf w}\) \(\newcommand{\xvec}{\mathbf x}\) \(\newcommand{\yvec}{\mathbf y}\) \(\newcommand{\zvec}{\mathbf z}\) \(\newcommand{\rvec}{\mathbf r}\) \(\newcommand{\mvec}{\mathbf m}\) \(\newcommand{\zerovec}{\mathbf 0}\) \(\newcommand{\onevec}{\mathbf 1}\) \(\newcommand{\real}{\mathbb R}\) \(\newcommand{\twovec}[2]{\left[\begin{array}{r}#1 \\ #2 \end{array}\right]}\) \(\newcommand{\ctwovec}[2]{\left[\begin{array}{c}#1 \\ #2 \end{array}\right]}\) \(\newcommand{\threevec}[3]{\left[\begin{array}{r}#1 \\ #2 \\ #3 \end{array}\right]}\) \(\newcommand{\cthreevec}[3]{\left[\begin{array}{c}#1 \\ #2 \\ #3 \end{array}\right]}\) \(\newcommand{\fourvec}[4]{\left[\begin{array}{r}#1 \\ #2 \\ #3 \\ #4 \end{array}\right]}\) \(\newcommand{\cfourvec}[4]{\left[\begin{array}{c}#1 \\ #2 \\ #3 \\ #4 \end{array}\right]}\) \(\newcommand{\fivevec}[5]{\left[\begin{array}{r}#1 \\ #2 \\ #3 \\ #4 \\ #5 \\ \end{array}\right]}\) \(\newcommand{\cfivevec}[5]{\left[\begin{array}{c}#1 \\ #2 \\ #3 \\ #4 \\ #5 \\ \end{array}\right]}\) \(\newcommand{\mattwo}[4]{\left[\begin{array}{rr}#1 \amp #2 \\ #3 \amp #4 \\ \end{array}\right]}\) \(\newcommand{\laspan}[1]{\text{Span}\{#1\}}\) \(\newcommand{\bcal}{\cal B}\) \(\newcommand{\ccal}{\cal C}\) \(\newcommand{\scal}{\cal S}\) \(\newcommand{\wcal}{\cal W}\) \(\newcommand{\ecal}{\cal E}\) \(\newcommand{\coords}[2]{\left\{#1\right\}_{#2}}\) \(\newcommand{\gray}[1]{\color{gray}{#1}}\) \(\newcommand{\lgray}[1]{\color{lightgray}{#1}}\) \(\newcommand{\rank}{\operatorname{rank}}\) \(\newcommand{\row}{\text{Row}}\) \(\newcommand{\col}{\text{Col}}\) \(\renewcommand{\row}{\text{Row}}\) \(\newcommand{\nul}{\text{Nul}}\) \(\newcommand{\var}{\text{Var}}\) \(\newcommand{\corr}{\text{corr}}\) \(\newcommand{\len}[1]{\left|#1\right|}\) \(\newcommand{\bbar}{\overline{\bvec}}\) \(\newcommand{\bhat}{\widehat{\bvec}}\) \(\newcommand{\bperp}{\bvec^\perp}\) \(\newcommand{\xhat}{\widehat{\xvec}}\) \(\newcommand{\vhat}{\widehat{\vvec}}\) \(\newcommand{\uhat}{\widehat{\uvec}}\) \(\newcommand{\what}{\widehat{\wvec}}\) \(\newcommand{\Sighat}{\widehat{\Sigma}}\) \(\newcommand{\lt}{<}\) \(\newcommand{\gt}{>}\) \(\newcommand{\amp}{&}\) \(\definecolor{fillinmathshade}{gray}{0.9}\)Learning Objectives
- Define non-experimental research, distinguish it clearly from experimental research, and give several examples.
- Explain when a researcher might choose to conduct non-experimental research as opposed to experimental research.
What Is Non-Experimental Research?
Non-experimental research is research that lacks the manipulation of an independent variable. Rather than manipulating an independent variable, researchers conducting non-experimental research simply measure variables as they naturally occur (in the lab or real world).
Most researchers in psychology consider the distinction between experimental and non-experimental research to be an extremely important one. This is because although experimental research can provide strong evidence that changes in an independent variable cause differences in a dependent variable, non-experimental research generally cannot. As we will see, however, this inability to make causal conclusions does not mean that non-experimental research is less important than experimental research.
When to Use Non-Experimental Research
As we saw in the last chapter , experimental research is appropriate when the researcher has a specific research question or hypothesis about a causal relationship between two variables—and it is possible, feasible, and ethical to manipulate the independent variable. It stands to reason, therefore, that non-experimental research is appropriate—even necessary—when these conditions are not met. There are many times in which non-experimental research is preferred, including when:
- the research question or hypothesis relates to a single variable rather than a statistical relationship between two variables (e.g., How accurate are people’s first impressions?).
- the research question pertains to a non-causal statistical relationship between variables (e.g., is there a correlation between verbal intelligence and mathematical intelligence?).
- the research question is about a causal relationship, but the independent variable cannot be manipulated or participants cannot be randomly assigned to conditions or orders of conditions for practical or ethical reasons (e.g., does damage to a person’s hippocampus impair the formation of long-term memory traces?).
- the research question is broad and exploratory, or is about what it is like to have a particular experience (e.g., what is it like to be a working mother diagnosed with depression?).
Again, the choice between the experimental and non-experimental approaches is generally dictated by the nature of the research question. Recall the three goals of science are to describe, to predict, and to explain. If the goal is to explain and the research question pertains to causal relationships, then the experimental approach is typically preferred. If the goal is to describe or to predict, a non-experimental approach will suffice. But the two approaches can also be used to address the same research question in complementary ways. For example, Similarly, after his original study, Milgram conducted experiments to explore the factors that affect obedience. He manipulated several independent variables, such as the distance between the experimenter and the participant, the participant and the confederate, and the location of the study (Milgram, 1974)[1].
Types of Non-Experimental Research
Non-experimental research falls into three broad categories: cross-sectional research, correlational research, and observational research.
First, cross-sectional research involves comparing two or more pre-existing groups of people. What makes this approach non-experimental is that there is no manipulation of an independent variable and no random assignment of participants to groups. Imagine, for example, that a researcher administers the Rosenberg Self-Esteem Scale to 50 American college students and 50 Japanese college students. Although this “feels” like a between-subjects experiment, it is a cross-sectional study because the researcher did not manipulate the students’ nationalities. As another example, if we wanted to compare the memory test performance of a group of cannabis users with a group of non-users, this would be considered a cross-sectional study because for ethical and practical reasons we would not be able to randomly assign participants to the cannabis user and non-user groups. Rather we would need to compare these pre-existing groups which could introduce a selection bias (the groups may differ in other ways that affect their responses on the dependent variable). For instance, cannabis users are more likely to use more alcohol and other drugs and these differences may account for differences in the dependent variable across groups, rather than cannabis use per se.
Cross-sectional designs are commonly used by developmental psychologists who study aging and by researchers interested in sex differences. Using this design, developmental psychologists compare groups of people of different ages (e.g., young adults spanning from 18-25 years of age versus older adults spanning 60-75 years of age) on various dependent variables (e.g., memory, depression, life satisfaction). Of course, the primary limitation of using this design to study the effects of aging is that differences between the groups other than age may account for differences in the dependent variable. For instance, differences between the groups may reflect the generation that people come from (a cohort effect) rather than a direct effect of age. For this reason, longitudinal studies in which one group of people is followed as they age offer a superior means of studying the effects of aging. Once again, cross-sectional designs are also commonly used to study sex differences. Since researchers cannot practically or ethically manipulate the sex of their participants they must rely on cross-sectional designs to compare groups of men and women on different outcomes (e.g., verbal ability, substance use, depression). Using these designs researchers have discovered that men are more likely than women to suffer from substance abuse problems while women are more likely than men to suffer from depression. But, using this design it is unclear what is causing these differences. So, using this design it is unclear whether these differences are due to environmental factors like socialization or biological factors like hormones?
When researchers use a participant characteristic to create groups (nationality, cannabis use, age, sex), the independent variable is usually referred to as an experimenter-selected independent variable (as opposed to the experimenter-manipulated independent variables used in experimental research). Figure \(\PageIndex{1}\) shows data from a hypothetical study on the relationship between whether people make a daily list of things to do (a “to-do list”) and stress. Notice that it is unclear whether this is an experiment or a cross-sectional study because it is unclear whether the independent variable was manipulated by the researcher or simply selected by the researcher. If the researcher randomly assigned some participants to make daily to-do lists and others not to, then the independent variable was experimenter-manipulated and it is a true experiment. If the researcher simply asked participants whether they made daily to-do lists or not, then the independent variable it is experimenter-selected and the study is cross-sectional. The distinction is important because if the study was an experiment, then it could be concluded that making the daily to-do lists reduced participants’ stress. But if it was a cross-sectional study, it could only be concluded that these variables are statistically related. Perhaps being stressed has a negative effect on people’s ability to plan ahead. Or perhaps people who are more conscientious are more likely to make to-do lists and less likely to be stressed. The crucial point is that what defines a study as experimental or cross-sectional l is not the variables being studied, nor whether the variables are quantitative or categorical, nor the type of graph or statistics used to analyze the data. It is how the study is conducted.
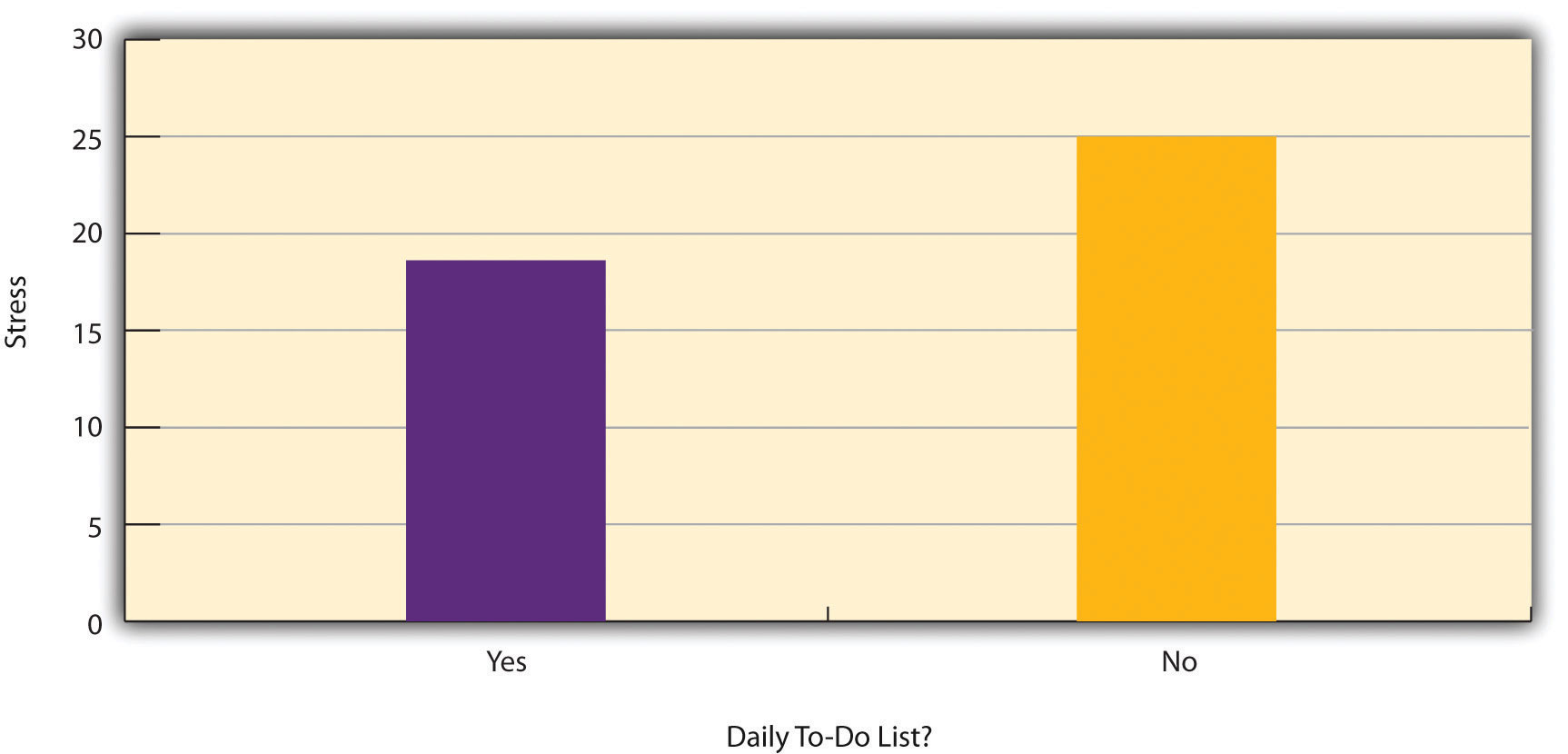
Second, the most common type of non-experimental research conducted in Psychology is correlational research. Correlational research is considered non-experimental because it focuses on the statistical relationship between two variables but does not include the manipulation of an independent variable. More specifically, in correlational research, the researcher measures two continuous variables with little or no attempt to control extraneous variables and then assesses the relationship between them. As an example, a researcher interested in the relationship between self-esteem and school achievement could collect data on students’ self-esteem and their GPAs to see if the two variables are statistically related. Correlational research is very similar to cross-sectional research, and sometimes these terms are used interchangeably. The distinction that will be made in this book is that, rather than comparing two or more pre-existing groups of people as is done with cross-sectional research, correlational research involves correlating two continuous variables (groups are not formed and compared).
Third, observational research is non-experimental because it focuses on making observations of behavior in a natural or laboratory setting without manipulating anything. Milgram’s original obedience study was non-experimental in this way. He was primarily interested in the extent to which participants obeyed the researcher when he told them to shock the confederate and he observed all participants performing the same task under the same conditions. The study by Loftus and Pickrell described at the beginning of this chapter is also a good example of observational research. The variable was whether participants “remembered” having experienced mildly traumatic childhood events (e.g., getting lost in a shopping mall) that they had not actually experienced but that the researchers asked them about repeatedly. In this particular study, nearly a third of the participants “remembered” at least one event. (As with Milgram’s original study, this study inspired several later experiments on the factors that affect false memories.
The types of research we have discussed so far are all quantitative, referring to the fact that the data consist of numbers that are analyzed using statistical techniques. But as you will learn in this chapter, many observational research studies are more qualitative in nature. In qualitative research , the data are usually nonnumerical and therefore cannot be analyzed using statistical techniques. Rosenhan’s observational study of the experience of people in a psychiatric ward was primarily qualitative. The data were the notes taken by the “pseudopatients”—the people pretending to have heard voices—along with their hospital records. Rosenhan’s analysis consists mainly of a written description of the experiences of the pseudopatients, supported by several concrete examples. To illustrate the hospital staff’s tendency to “depersonalize” their patients, he noted, “Upon being admitted, I and other pseudopatients took the initial physical examinations in a semi-public room, where staff members went about their own business as if we were not there” (Rosenhan, 1973, p. 256)[2]. Qualitative data has a separate set of analysis tools depending on the research question. For example, thematic analysis would focus on themes that emerge in the data or conversation analysis would focus on the way the words were said in an interview or focus group.
Internal Validity Revisited
Recall that internal validity is the extent to which the design of a study supports the conclusion that changes in the independent variable caused any observed differences in the dependent variable. Figure \(\PageIndex{2}\) shows how experimental, quasi-experimental, and non-experimental (correlational) research vary in terms of internal validity. Experimental research tends to be highest in internal validity because the use of manipulation (of the independent variable) and control (of extraneous variables) help to rule out alternative explanations for the observed relationships. If the average score on the dependent variable in an experiment differs across conditions, it is quite likely that the independent variable is responsible for that difference. Non-experimental (correlational) research is lowest in internal validity because these designs fail to use manipulation or control. Quasi-experimental research (which will be described in more detail in a subsequent chapter) is in the middle because it contains some, but not all, of the features of a true experiment. For instance, it may fail to use random assignment to assign participants to groups or fail to use counterbalancing to control for potential order effects. Imagine, for example, that a researcher finds two similar schools, starts an anti-bullying program in one, and then finds fewer bullying incidents in that “treatment school” than in the “control school.” While a comparison is being made with a control condition, the lack of random assignment of children to schools could still mean that students in the treatment school differed from students in the control school in some other way that could explain the difference in bullying (e.g., there may be a selection effect).

Notice also in Figure \(\PageIndex{2}\) that there is some overlap in the internal validity of experiments, quasi-experiments, and correlational studies. For example, a poorly designed experiment that includes many confounding variables can be lower in internal validity than a well-designed quasi-experiment with no obvious confounding variables. Internal validity is also only one of several validities that one might consider, as noted in Chapter 5.
Key Takeaways
- Non-experimental research is research that lacks the manipulation of an independent variable.
- There are two broad types of non-experimental research. Correlational research that focuses on statistical relationships between variables that are measured but not manipulated, and observational research in which participants are observed and their behavior is recorded without the researcher interfering or manipulating any variables.
- In general, experimental research is high in internal validity, correlational research is low in internal validity, and quasi-experimental research is in between.
Exercises
- Discussion: For each of the following studies, decide which type of research design it is and explain why.
- A researcher conducts detailed interviews with unmarried teenage fathers to learn about how they feel and what they think about their role as fathers and summarizes their feelings in a written narrative.
- A researcher measures the impulsivity of a large sample of drivers and looks at the statistical relationship between this variable and the number of traffic tickets the drivers have received.
- A researcher randomly assigns patients with low back pain either to a treatment involving hypnosis or to a treatment involving exercise. She then measures their level of low back pain after 3 months.
- A college instructor gives weekly quizzes to students in one section of his course but no weekly quizzes to students in another section to see whether this has an effect on their test performance.
References
- Milgram, S. (1974). Obedience to authority: An experimental view. New York, NY: Harper & Row.
- Rosenhan, D. L. (1973). On being sane in insane places. Science, 179, 250–258.