Let us conclude this chapter with a brief discussion of the role that hypothesis testing plays within a given strand of research, i.e., within the context of a set of research projects dealing with a particular research question (or a set of such questions), starting, again, with Karl Popper. Popper is sometimes portrayed as advocating an almost mindless version of falsificationism, where researchers randomly pull hypotheses out of thin air and test them until they are falsified, then start again with a new randomly invented hypothesis.
Popper’s actual discussions are closer to actual scientific practice. It is true that in terms of scientific logic, the only requirement of a hypothesis is that it is testable (i.e., falsifiable), but in scientific practice, it must typically meet two additional criteria: first, it must be a potentially insightful explanation of a particular research problem, and second, it must take into account previous research (if such research exists). It is also true that falsification is central to Popperian research logic, but not as a mindless slashing of ideas, but as a process of error elimination. Popper describes the entire process using the following schematic representation (Popper 1970: 3):
(31) P1 → TT → EE → P2
In this schema, P1 stands for a research question (a “problem”), TT stands for a hypothesis (a “tentative theory”), EE for the attempt to falsify the hypothesis (“error elimination”) by testing, but also by “critical discussion”, and P2 stands for new or additional problems and research questions arising from the falsification process.4 Popper also acknowledges that it is good research practice to entertain several hypotheses at once, if there is more than one promising explanation for a problem situation, expanding his formula as follows:
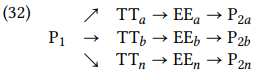
He explicitly acknowledges that falsification, while central, is not the only criterion by which science proceeds: if there are several unfalsified hypotheses, we may also assess them based on which promises the most insightful explanation or which produces the most interesting additional hypotheses (Popper 1970: 3).
Crucially, (31) and (32) suggest a cyclic and incremental approach to research: the status quo in a given field is the result of a long process of producing new hypotheses and eliminating errors, and it will, in turn, serve as a basis for more new hypotheses (and more errors which need to be eliminated). This incremental cyclicity can actually be observed in scientific disciplines. In some, like physics or psychology, researchers make this very explicit, publishing research in the form of series of experiments attempting to falsify certain existing hypotheses and corroborating others, typically building on earlier experiments by others or themselves and closing with open questions and avenues of future research. In other disciplines, like the more humanities-leaning social sciences including (corpus) linguistics, the cycle is typically less explicit, but viewed from a distance, researchers also follow this procedure, summarizing the ideas of previous authors (sometimes to epic lengths) and then adding more or less substantial data and arguments of their own.
Fleshing out Popper’s basic schema in (31) above, drawing together the points discussed in this and the previous chapter, we can represent this cycle as shown in Figure 3.1.
Research begins with a general question – something that intrigues an individual or a group of researchers. The part of reality to which this question pertains is then modeled, i.e., described in terms of theoretical constructs, enabling us to formulate, first, a more specific research question, and often, second, a hypothesis.
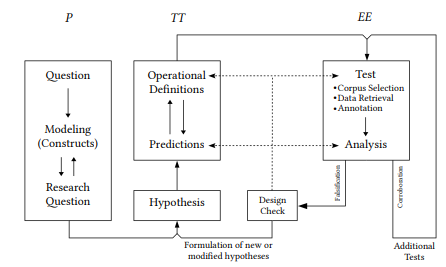
Figure 3.1: The scientific research cycle
There is nothing automatic about these steps – they are typically characterized by lengthy critical discussion, false starts or wild speculation, until testable hypotheses emerge (in some disciplines, this stage has not yet been, and in some cases probably never will be reached). Next, predictions must be derived, requiring operational definitions of the constructs posited previously. This may require some back and forth between formulating predictions and providing sufficiently precise operationalizations.
Next, the predictions must be tested – in the case of corpus linguistics, corpora must be selected and data must be retrieved and annotated, something we will discuss in detail in the next chapter. Then the data are analyzed with respect to the hypothesis. If they corroborate the hypothesis (or at least fail to falsify it), this is not the end of the process: with Popper, we should only begin to accept evidence as corroborating when it emerges from repeated attempts to falsify the hypothesis. Thus, additional tests must be, and typically are, devised. If the results of any test falsify the hypothesis, this does not, of course, lead to its immediate rejection. After all, we have typically arrived at our hypothesis based on good arguments, and so researchers will typically perform what we could call a “design check” on their experiment, looking closely at their predictions to see if they really follow from the hypothesis, the operational definitions to see whether they are reliable and valid with respect to the constructs they represent, and the test itself to determine whether there are errors or confounding variables in the data selection and analysis. If potential problems are found, they will be fixed and the test will be repeated. Only if it fails repeatedly will researchers abandon (or modify) the hypothesis.
The repeated testing, and especially the modification of a hypothesis is inherently dangerous, as we might be so attached to our hypothesis that we will keep testing it long after we should have given it up, or that we will try to save it by changing it just enough that our test will no longer falsify it, or by making it completely untestable (cf. Popper 1963: 37). This must, of course, be avoided, but so must throwing out a hypothesis, or an entire model, on the basis of a single falsification event. Occasionally, especially in half-mature disciplines like linguistics, models morph into competing schools of thought, each vigorously defended by its adherents even in the face of a growing number of phenomena that they fail to account for. In such cases, a radical break in the research cycles within these models may be necessary to make any headway at all – a so-called “paradigm shifts” occurs. This means that researchers abandon the current model wholesale and start from scratch based on different initial assumptions (see Kuhn 1962). Corpus linguistics with its explicit recognition that generalizations about the language system can and must be deduced from language usage may present such a paradigm shift with respect to the intuition-driven generative models.
Finally, note that the scientific research cycle is not only incremental, with each new hypothesis and each new test building on previous research, but that it is also collaborative, with one researcher or group of researchers picking up where another left off. This collaborative nature of research requires researchers to be maximally transparent with respect to their research designs, laying open their data and methods in sufficient detail for other researchers to understand exactly what prediction was tested, how the constructs in question were operationalized, how data were retrieved and analyzed. Again, this is the norm in disciplines like experimental physics and psychology, but not so much so in the more humanities-leaning disciplines, which tend to put the focus on ideas and arguments rather than methods. We will deal with data retrieval and annotation in the next chapter and return to the issue of methodological transparency at the end of it.
4 Of course, Popper did not invent, or claim to have invented, this procedure. He was simply explicating what he thought successful scientists were, and ought to be, doing (Rudolph (2005) traces the explicit recognition of this procedure to John Dewey’s still very readable How we think (Dewey 1910), which contains insightful illustrations).