10.6: Understanding Complex Systems
- Page ID
- 77005
\( \newcommand{\vecs}[1]{\overset { \scriptstyle \rightharpoonup} {\mathbf{#1}} } \)
\( \newcommand{\vecd}[1]{\overset{-\!-\!\rightharpoonup}{\vphantom{a}\smash {#1}}} \)
\( \newcommand{\id}{\mathrm{id}}\) \( \newcommand{\Span}{\mathrm{span}}\)
( \newcommand{\kernel}{\mathrm{null}\,}\) \( \newcommand{\range}{\mathrm{range}\,}\)
\( \newcommand{\RealPart}{\mathrm{Re}}\) \( \newcommand{\ImaginaryPart}{\mathrm{Im}}\)
\( \newcommand{\Argument}{\mathrm{Arg}}\) \( \newcommand{\norm}[1]{\| #1 \|}\)
\( \newcommand{\inner}[2]{\langle #1, #2 \rangle}\)
\( \newcommand{\Span}{\mathrm{span}}\)
\( \newcommand{\id}{\mathrm{id}}\)
\( \newcommand{\Span}{\mathrm{span}}\)
\( \newcommand{\kernel}{\mathrm{null}\,}\)
\( \newcommand{\range}{\mathrm{range}\,}\)
\( \newcommand{\RealPart}{\mathrm{Re}}\)
\( \newcommand{\ImaginaryPart}{\mathrm{Im}}\)
\( \newcommand{\Argument}{\mathrm{Arg}}\)
\( \newcommand{\norm}[1]{\| #1 \|}\)
\( \newcommand{\inner}[2]{\langle #1, #2 \rangle}\)
\( \newcommand{\Span}{\mathrm{span}}\) \( \newcommand{\AA}{\unicode[.8,0]{x212B}}\)
\( \newcommand{\vectorA}[1]{\vec{#1}} % arrow\)
\( \newcommand{\vectorAt}[1]{\vec{\text{#1}}} % arrow\)
\( \newcommand{\vectorB}[1]{\overset { \scriptstyle \rightharpoonup} {\mathbf{#1}} } \)
\( \newcommand{\vectorC}[1]{\textbf{#1}} \)
\( \newcommand{\vectorD}[1]{\overrightarrow{#1}} \)
\( \newcommand{\vectorDt}[1]{\overrightarrow{\text{#1}}} \)
\( \newcommand{\vectE}[1]{\overset{-\!-\!\rightharpoonup}{\vphantom{a}\smash{\mathbf {#1}}}} \)
\( \newcommand{\vecs}[1]{\overset { \scriptstyle \rightharpoonup} {\mathbf{#1}} } \)
\( \newcommand{\vecd}[1]{\overset{-\!-\!\rightharpoonup}{\vphantom{a}\smash {#1}}} \)
Social traps, such as the tragedy of the commons or time-delay, help to explain much of the unsustainable resource use currently taking place. Yet even without these traps, our fundamental misunderstanding of social, economic, and ecological systems tends to promote poor decision making and complacency regarding the natural systems that support us. The term social-ecological system is used to indicate the interactions between social, economic, and ecological systems. These systems are complex, meaning they are dynamic (rather than static) and characterized by web-like causal connections (rather than linear causal chains). Our failure to acknowledge and understand these characteristics causes us to be surprised by their unexpected behaviours. In this section, we will look at each of these characteristics of complexity.
Dynamic Systems
We tend to think of social-ecological systems as static systems that exhibit linear behaviour. A bicycle exhibits this behaviour. If you pedal at a certain rate, the bicycle will move at a corresponding speed. If you double your rate of pedalling, the bicycle will move at roughly twice the original speed. This is called a linear response. Now imagine a bicycle that would take off like a rocket if you doubled your pedalling rate or one that would some days barely respond to pedalling at all. This is closer to how complex systems behave. With a basic understanding of complex systems this behaviour can be explained and to a certain extent even predicted.
Erling Moxnes (2000) performed an interesting experiment to show how our inability to understand the dynamics of complex systems can contribute to the collapse of a resource. Moxnes designed a model of a fishery based on the population dynamics described in the textbox above. He then had study participants (including fishers and fishery managers) manage this simulated fishery. Each year a participant could decide whether or not to add a ship to the fishing fleet. The participant would then receive data regarding the number of fish caught that year and, based on that information, make a decision about adding another ship the following year.
Two characteristics about this exercise are relevant to our discussion of social traps. First, the simulated fishery was privately owned. Participants did not have to worry about someone else catching the fish that they left to reproduce. Second, the participants themselves were rewarded (i.e. paid) based on their success in running a sustainable fishery based on an infinite time horizon. In other words, the higher immediate payoff to the participants came if they were able to maintain a high reproductive rate in the fishery far into the future. By setting the game up this way, Moxnes effectively eliminated the potential for tragedy of the commons or time-delay traps, presumably taking away the most difficult aspects of managing real-world fisheries.
Despite these advantages, the median fleet size built by Moxnes’ participants was almost double the size required to maximize sustainable yield. Even without the social traps described above, the participants overfished their fisheries. Perhaps even more interesting than the participants’ poor performances were their responses to this and other similar simulations. Many were dubious of the results, suggesting an error in the model itself or attributing their performance to factors outside the parameters of the model (e.g. disease).
The difficulty for these participants stemmed from their assumption that the fishery was a static, rather than a dynamic system. Moxnes explains that the participants assumed there was a set rate of growth, say 1000 fish per year. They proceeded to increase their fleet size, assuming that a decrease in catch would indicate that they had found the growth rate. They did not realize that growth rate is a moving target, which decreases as the population size becomes smaller. By the time participants observed a decrease in catch, they had already decreased the population significantly, causing a severe reduction in the population’s ability to make more fish.
Moxnes’ findings apply to far more than fisheries. Social, economic, and ecological systems are all complex, dynamic systems. Because of our tendency to view these systems as static, we are consistently surprised by their behaviour in the same way that Moxnes’ participants were surprised by the response of the model. Consider, for example, the phenomenon of positive or reinforcing feedback, where a small change leads to bigger and bigger change. The build-up of nuclear arms during the Cold War is an oft-cited example. The United States developed a small arsenal of nuclear weapons. The Soviet Union, viewing this as a threat, developed their own arsenal in response. The United States viewed this response as a threat and added to their arsenal, and the Soviet Union behaved likewise. This cycle continued until the two countries had enough nuclear arms to blow up the Earth many times over—a situation that was costly and dangerous to both countries.
To see how reinforcing feedback works in the context of natural resources, we can return to the example of the green revolution. Scientists responded to the perceived threat of the human population becoming too large to feed by increasing our ability to produce food. Forgetting for the moment the objections raised regarding the agricultural methods used to increase food production, we can focus on the problem with the logic of this solution in a dynamic system. If the amount of food needed to feed the world population were a static figure (as is assumed in the slogan ‘feeding the world’), then increasing food supply alone would have indeed solved the problem. However, as we have seen with fish populations, population growth involves a complex and dynamic system.
The reinforcing loop that illustrates this pattern is shown in Figure 10.3. To understand the dynamics of the system, one must simply follow the arrows in the loop. Reproduction leads to an increase in population size, which leads to an increase in reproduction, which leads to an increase in population size. And on it goes round and round the loop. The result of this feedback loop in populations is the exponential growth described earlier in the chapter (Figure 10.4). This pattern of growth can be seen in populations of many species. However, reinforcing loops are constrained by a balancing loop. The growth of populations is typically constrained by available resources, as shown in the fishery example in Figure 10.2.
This pattern is basic ecology, but it was not a part of the solution offered by the green revolution. Increasing the global food supply further increased the global population. In the 1960s, people were worried about feeding a population of four billion. In the next few decades, we will be concerned about feeding a population of nine to 10 billion. In short, increasing food production made it possible to go around the reinforcing feedback loop of Figure 10.3 several more times, but it did not address the fundamental problem. A more holistic approach to the problem might have included measures for addressing population growth (e.g. family planning programmes). By choosing to limit our reproduction rates before we are forced to by resource constraints, we can address the root of the famine and suffering that the scientists of the green revolution intended to address.

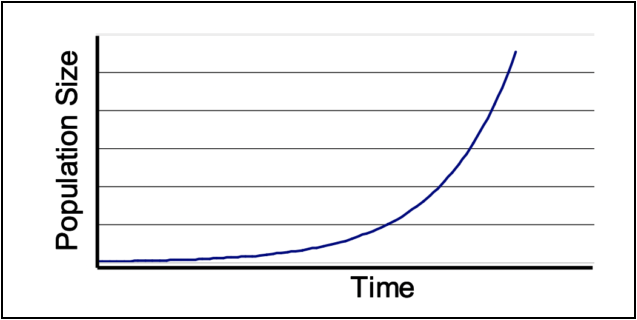
Interconnectedness
In addition to being dynamic, complex systems are characterized by a high level of interdependence between parts. When we analyse a process, we tend to think in linear terms with series of events making causal chains, but in complex systems one cause can have many effects that move through a system. Ecologists often observe this type of behaviour, in which the loss of one species causes major changes to the entire system. For example, fishers, observing otters eating fish, used to view them as competitors for fish near a kelp forest. Now, however, scientists know that the absence of otters in a kelp forest system can lead to the loss of the entire forest, including the fish.
In terms of natural resources, particularly renewable resources, this interconnectivity can mean that activity regarding one resource leads to difficulties with others as well. We have already seen how activity in states along the Mississippi River affects resources in the Gulf of Mexico. Examples abound. One area that has received greater attention recently is the connection between coastal forests and marine systems. Each of these systems provides important resources and services. Only recently have resource managers looked closely at how these systems interact. For example, loss of coastal forests can significantly increase the amount of nutrients and sedimentation entering nearby coral reefs (Caddy & Bakun, 1994; Humborg et al., 2000). In short, scarcity in one resource can cause scarcity in others as well. In addition, environmental degradation taking place on a global scale (see Chapter 9 and Chapter 12) can cause severe reductions in available living resources.